The Different 'Types of Data'
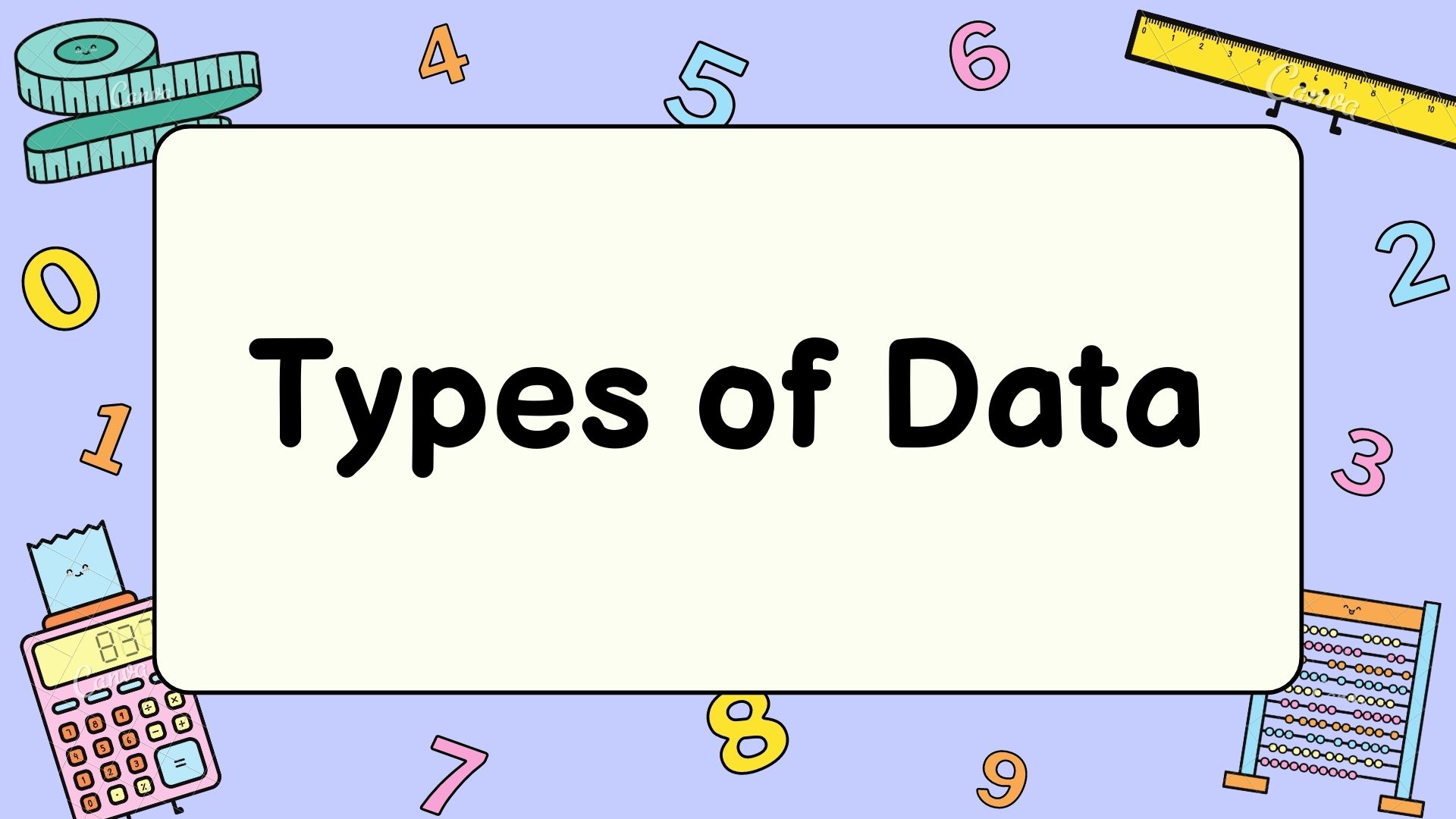
Welcome to this statistics blog! In this, we will explore the various types of data commonly encountered in statistical analysis. Understanding these different types is crucial for conducting accurate analyses and drawing meaningful conclusions from your data. Let's dive in!
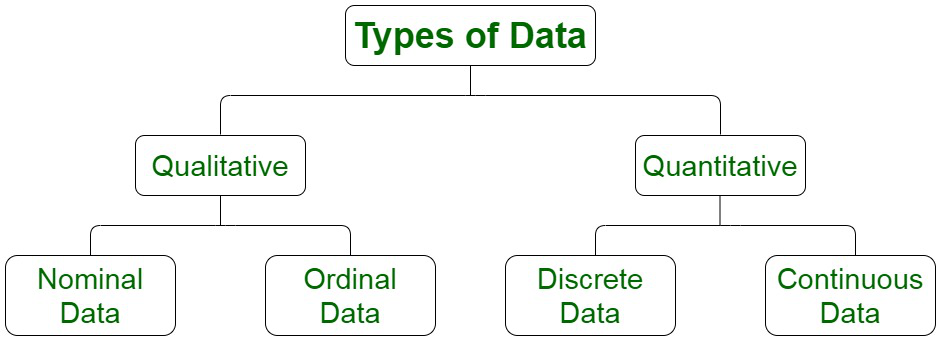
Numerical Data / Quantitative Data
Numerical data, also known as quantitative data, consists of numerical values that can be measured or counted. It can further be categorized into two subtypes:
- Discrete Data: Discrete data represents whole numbers or counts that have specific values. For example, the number of cars in a parking lot or the number of children in a family.
- Continuous Data: Continuous data represents measurements that can take any value within a range. Examples include height, weight, temperature, or time. Continuous data is typically obtained through instruments or measurements.
Example: The number of goals scored by a soccer team in a match, or the number of students in a classroom.
Example: The number of goals scored by a soccer team in a match, or the number of students in a classroom.
Categorical Data / Qualitative Data
Numerical data, also known as quantitative data, consists of numerical values that can be measured or counted. It can further be categorized into two subtypes:
- Nominal Data: Nominal data represents data without any natural order or ranking. Examples include gender (male or female), eye color (blue, green, or brown), or favorite sports teams.
- Ordinal Data: Ordinal data represents data with a natural order or ranking. It involves categories that have a relative position or value. Examples include survey responses with options like "strongly disagree," "disagree," "neutral," "agree," and "strongly agree."
Example: The type of car models owned by individuals (e.g., Sedan, SUV, Truck), or the political affiliation of voters (e.g., Democrat, Republican, Independent).
Example: Customer satisfaction levels rated as "very unsatisfied," "unsatisfied," "neutral," "satisfied," or "very satisfied," or educational levels categorized as "high school," "bachelor's degree," "master's degree," or "Ph.D."
Conclusion
Understanding the different types of data is fundamental to statistics and data analysis. Whether you are working with numerical data, categorical data, time series data, recognizing the characteristics of each type will help you choose appropriate statistical techniques and interpret your results accurately.
That's all for this blog, See you next time!